Difficulty of âlearningâ rare instances
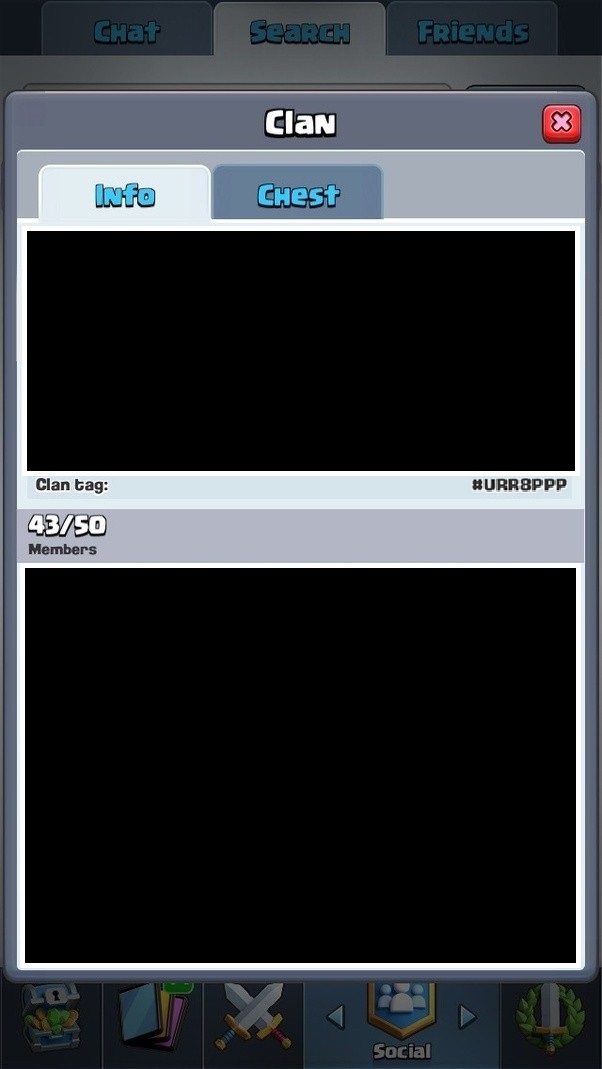

up vote
8
down vote
favorite
Is there any result showing that models (say SVM, Neural-Net, kNN, etc) will have difficulty in learning "rare" instances/tail phenomena?
machine-learning lg.learning pac-learning
add a comment |Â
up vote
8
down vote
favorite
Is there any result showing that models (say SVM, Neural-Net, kNN, etc) will have difficulty in learning "rare" instances/tail phenomena?
machine-learning lg.learning pac-learning
I think you accepted an answer too quickly -- it's a good answer, but there could be more possibilities out there as well.
â usul
Aug 8 at 8:35
@usul thanks for the comment. Do you have any further suggestion? Would be happy to hear additional thoughts.
â Daniel
Aug 8 at 14:13
add a comment |Â
up vote
8
down vote
favorite
up vote
8
down vote
favorite
Is there any result showing that models (say SVM, Neural-Net, kNN, etc) will have difficulty in learning "rare" instances/tail phenomena?
machine-learning lg.learning pac-learning
Is there any result showing that models (say SVM, Neural-Net, kNN, etc) will have difficulty in learning "rare" instances/tail phenomena?
machine-learning lg.learning pac-learning
asked Aug 7 at 15:53
Daniel
484312
484312
I think you accepted an answer too quickly -- it's a good answer, but there could be more possibilities out there as well.
â usul
Aug 8 at 8:35
@usul thanks for the comment. Do you have any further suggestion? Would be happy to hear additional thoughts.
â Daniel
Aug 8 at 14:13
add a comment |Â
I think you accepted an answer too quickly -- it's a good answer, but there could be more possibilities out there as well.
â usul
Aug 8 at 8:35
@usul thanks for the comment. Do you have any further suggestion? Would be happy to hear additional thoughts.
â Daniel
Aug 8 at 14:13
I think you accepted an answer too quickly -- it's a good answer, but there could be more possibilities out there as well.
â usul
Aug 8 at 8:35
I think you accepted an answer too quickly -- it's a good answer, but there could be more possibilities out there as well.
â usul
Aug 8 at 8:35
@usul thanks for the comment. Do you have any further suggestion? Would be happy to hear additional thoughts.
â Daniel
Aug 8 at 14:13
@usul thanks for the comment. Do you have any further suggestion? Would be happy to hear additional thoughts.
â Daniel
Aug 8 at 14:13
add a comment |Â
1 Answer
1
active
oldest
votes
up vote
10
down vote
accepted
In the classic PAC learning (i.e., classification) model, rare instances are not a problem. This is because the learner's test points are assumed to come from the same distribution as the training data. Thus, if a region of space is so sparse as to be poorly represented in the training sample, its probability of appearing during the test phase is low.
You'll need a different learning model, which explicitly looks at type-I and type-II errors, or perhaps some combined precision-recall score. Here again, I don't think there are any results indicating that a specific class of algorithms is particularly poorly suited for this task, but I could be wrong.
The closest I can think of is sensitivity to outliers --- AdaBoost is known to have this property, for example.
add a comment |Â
1 Answer
1
active
oldest
votes
1 Answer
1
active
oldest
votes
active
oldest
votes
active
oldest
votes
up vote
10
down vote
accepted
In the classic PAC learning (i.e., classification) model, rare instances are not a problem. This is because the learner's test points are assumed to come from the same distribution as the training data. Thus, if a region of space is so sparse as to be poorly represented in the training sample, its probability of appearing during the test phase is low.
You'll need a different learning model, which explicitly looks at type-I and type-II errors, or perhaps some combined precision-recall score. Here again, I don't think there are any results indicating that a specific class of algorithms is particularly poorly suited for this task, but I could be wrong.
The closest I can think of is sensitivity to outliers --- AdaBoost is known to have this property, for example.
add a comment |Â
up vote
10
down vote
accepted
In the classic PAC learning (i.e., classification) model, rare instances are not a problem. This is because the learner's test points are assumed to come from the same distribution as the training data. Thus, if a region of space is so sparse as to be poorly represented in the training sample, its probability of appearing during the test phase is low.
You'll need a different learning model, which explicitly looks at type-I and type-II errors, or perhaps some combined precision-recall score. Here again, I don't think there are any results indicating that a specific class of algorithms is particularly poorly suited for this task, but I could be wrong.
The closest I can think of is sensitivity to outliers --- AdaBoost is known to have this property, for example.
add a comment |Â
up vote
10
down vote
accepted
up vote
10
down vote
accepted
In the classic PAC learning (i.e., classification) model, rare instances are not a problem. This is because the learner's test points are assumed to come from the same distribution as the training data. Thus, if a region of space is so sparse as to be poorly represented in the training sample, its probability of appearing during the test phase is low.
You'll need a different learning model, which explicitly looks at type-I and type-II errors, or perhaps some combined precision-recall score. Here again, I don't think there are any results indicating that a specific class of algorithms is particularly poorly suited for this task, but I could be wrong.
The closest I can think of is sensitivity to outliers --- AdaBoost is known to have this property, for example.
In the classic PAC learning (i.e., classification) model, rare instances are not a problem. This is because the learner's test points are assumed to come from the same distribution as the training data. Thus, if a region of space is so sparse as to be poorly represented in the training sample, its probability of appearing during the test phase is low.
You'll need a different learning model, which explicitly looks at type-I and type-II errors, or perhaps some combined precision-recall score. Here again, I don't think there are any results indicating that a specific class of algorithms is particularly poorly suited for this task, but I could be wrong.
The closest I can think of is sensitivity to outliers --- AdaBoost is known to have this property, for example.
answered Aug 7 at 17:59
Aryeh
4,6561632
4,6561632
add a comment |Â
add a comment |Â
Sign up or log in
StackExchange.ready(function ()
StackExchange.helpers.onClickDraftSave('#login-link');
var $window = $(window),
onScroll = function(e)
var $elem = $('.new-login-left'),
docViewTop = $window.scrollTop(),
docViewBottom = docViewTop + $window.height(),
elemTop = $elem.offset().top,
elemBottom = elemTop + $elem.height();
if ((docViewTop elemBottom))
StackExchange.using('gps', function() StackExchange.gps.track('embedded_signup_form.view', location: 'question_page' ); );
$window.unbind('scroll', onScroll);
;
$window.on('scroll', onScroll);
);
Sign up using Google
Sign up using Facebook
Sign up using Email and Password
Post as a guest
StackExchange.ready(
function ()
StackExchange.openid.initPostLogin('.new-post-login', 'https%3a%2f%2fcstheory.stackexchange.com%2fquestions%2f41334%2fdifficulty-of-learning-rare-instances%23new-answer', 'question_page');
);
Post as a guest
Sign up or log in
StackExchange.ready(function ()
StackExchange.helpers.onClickDraftSave('#login-link');
var $window = $(window),
onScroll = function(e)
var $elem = $('.new-login-left'),
docViewTop = $window.scrollTop(),
docViewBottom = docViewTop + $window.height(),
elemTop = $elem.offset().top,
elemBottom = elemTop + $elem.height();
if ((docViewTop elemBottom))
StackExchange.using('gps', function() StackExchange.gps.track('embedded_signup_form.view', location: 'question_page' ); );
$window.unbind('scroll', onScroll);
;
$window.on('scroll', onScroll);
);
Sign up using Google
Sign up using Facebook
Sign up using Email and Password
Post as a guest
Sign up or log in
StackExchange.ready(function ()
StackExchange.helpers.onClickDraftSave('#login-link');
var $window = $(window),
onScroll = function(e)
var $elem = $('.new-login-left'),
docViewTop = $window.scrollTop(),
docViewBottom = docViewTop + $window.height(),
elemTop = $elem.offset().top,
elemBottom = elemTop + $elem.height();
if ((docViewTop elemBottom))
StackExchange.using('gps', function() StackExchange.gps.track('embedded_signup_form.view', location: 'question_page' ); );
$window.unbind('scroll', onScroll);
;
$window.on('scroll', onScroll);
);
Sign up using Google
Sign up using Facebook
Sign up using Email and Password
Post as a guest
Sign up or log in
StackExchange.ready(function ()
StackExchange.helpers.onClickDraftSave('#login-link');
var $window = $(window),
onScroll = function(e)
var $elem = $('.new-login-left'),
docViewTop = $window.scrollTop(),
docViewBottom = docViewTop + $window.height(),
elemTop = $elem.offset().top,
elemBottom = elemTop + $elem.height();
if ((docViewTop elemBottom))
StackExchange.using('gps', function() StackExchange.gps.track('embedded_signup_form.view', location: 'question_page' ); );
$window.unbind('scroll', onScroll);
;
$window.on('scroll', onScroll);
);
Sign up using Google
Sign up using Facebook
Sign up using Email and Password
Sign up using Google
Sign up using Facebook
Sign up using Email and Password
I think you accepted an answer too quickly -- it's a good answer, but there could be more possibilities out there as well.
â usul
Aug 8 at 8:35
@usul thanks for the comment. Do you have any further suggestion? Would be happy to hear additional thoughts.
â Daniel
Aug 8 at 14:13